Decoding AI: Exploring the Core Technologies Shaping Tomorrow
Read Time 21 mins | Written by: Sarah Grace Hays
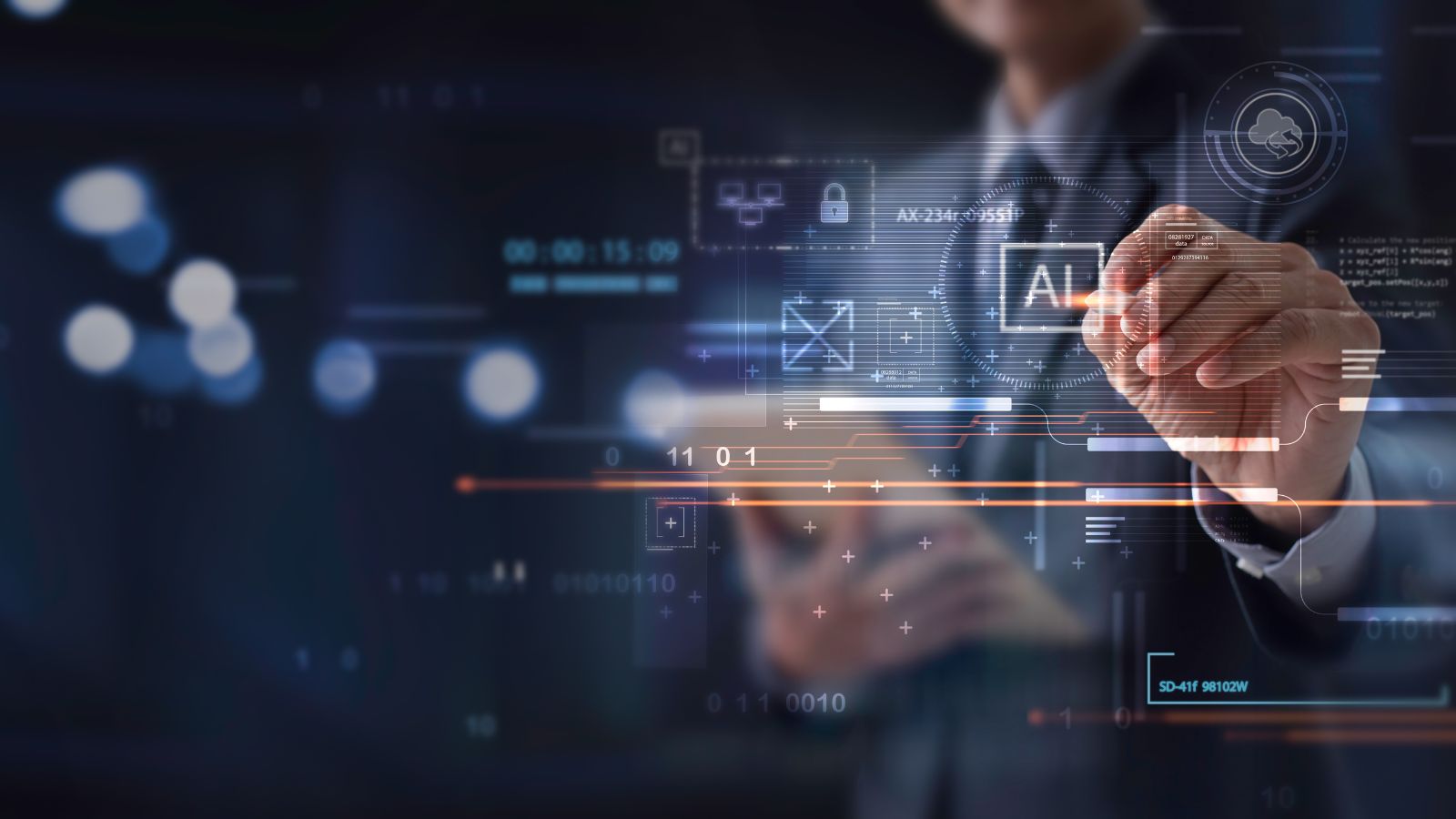
Practically every day, there is a new headline about AI. Whether it be rumors about an OpenAI search engine or reports on the economic benefits of AI, there is a constant flow of information and innovation around AI. News organizations like CNN, The Washington Post, The New York Times, and Fox have created dedicated AI news pages, and sites like TechCrunch publish multiple articles about AI daily.
The influx of focus on AI is no surprise. Statista reported that in 2023, the global AI market size was close to $208 billion, and the market is expected to reach nearly $2 trillion by 2030. AI is exciting, and seemingly everyone knows it.
The growth of AI tools is ascending every day, and all data indicates it will only continue to climb. A few important AI technologies foster this inevitable growth you must understand as a baseline. So, let's review some foundational technologies for the awesome AI tools we see today.
Automated Machine Learning
Machine learning is a branch of artificial intelligence (AI) and computer science that focuses on using data and algorithms to enable AI to imitate how humans learn, gradually improving its accuracy. This process is done by humans and can be incredibly time-consuming.
Automated machine learning automates the time-consuming, iterative machine learning model development tasks. It allows data scientists, analysts, and developers to build ML models with high scale, efficiency, and productivity, all while sustaining model quality.
AutoML aims to create a system to build its end-to-end machine-learning models. Machine learning has the potential to solve a wide range of problems, from image recognition to natural language processing, and AutoML democratizes this and makes it more widely accessible.
Simply put, AutoML initiates with training data comprising attributes paired with a target variable, representing what you aim to predict. Algorithms analyze this data, generating models most accurately capturing the correlation between attributes and the target. The optimal model is determined through evaluations conducted on unseen data held aside for testing purposes.
However, AutoML encompasses various techniques, and the process may differ based on the specific platform or tool used.
There are serious benefits to AutoML:
- Minimization of human error
- Boosted efficiency and productivity
- Constant innovation and adaptation
Generative AI
Generative AI is a type of artificial intelligence technology that can generate different types of content from audio to text to imagery. Generative AI is essentially a machine learning model that is trained to create new data rather than make predictions about a data set.
In recent years increasingly user-friendly GenAI systems have made this technology more popular.
According to PwC, fifty-four percent of companies had uses generative AI in their business by November 2023, just one year after the release of ChatGPT. Additionally, Deloitte reported 62% of surveyed companies had integrated generative AI technologies into their operations within the first year of adoption.
Generative AI is one of the older forms of AI, dating back to the Markov Chain. Markov models have been used for text-prediction tasks. You might imagine how your phone suggests the next word in your sentence when texting someone.
Today's generative AI is trained on an enormous amount of data.
Early versions of generative AI were all about sending data through APIs or complex processes. Developers had to wrestle with fancy tools and write code using languages like Python.
Now, pioneers in generative AI are creating better user interfaces that let you express your requests in plain language. After you get that first response, you can fine-tune the results by giving feedback on style and tone, making the generated content yours.
For instance, OpenAI recently released a new version of ChatGPT featuring enhanced user interfaces and real-time feedback mechanisms. This allows users to easily customize generated content according to their preferences.
For fun here is an image I had OpenAI’s DALL-E generate using the prompt: “As many cats as possible in giant office working hard at their computers”:
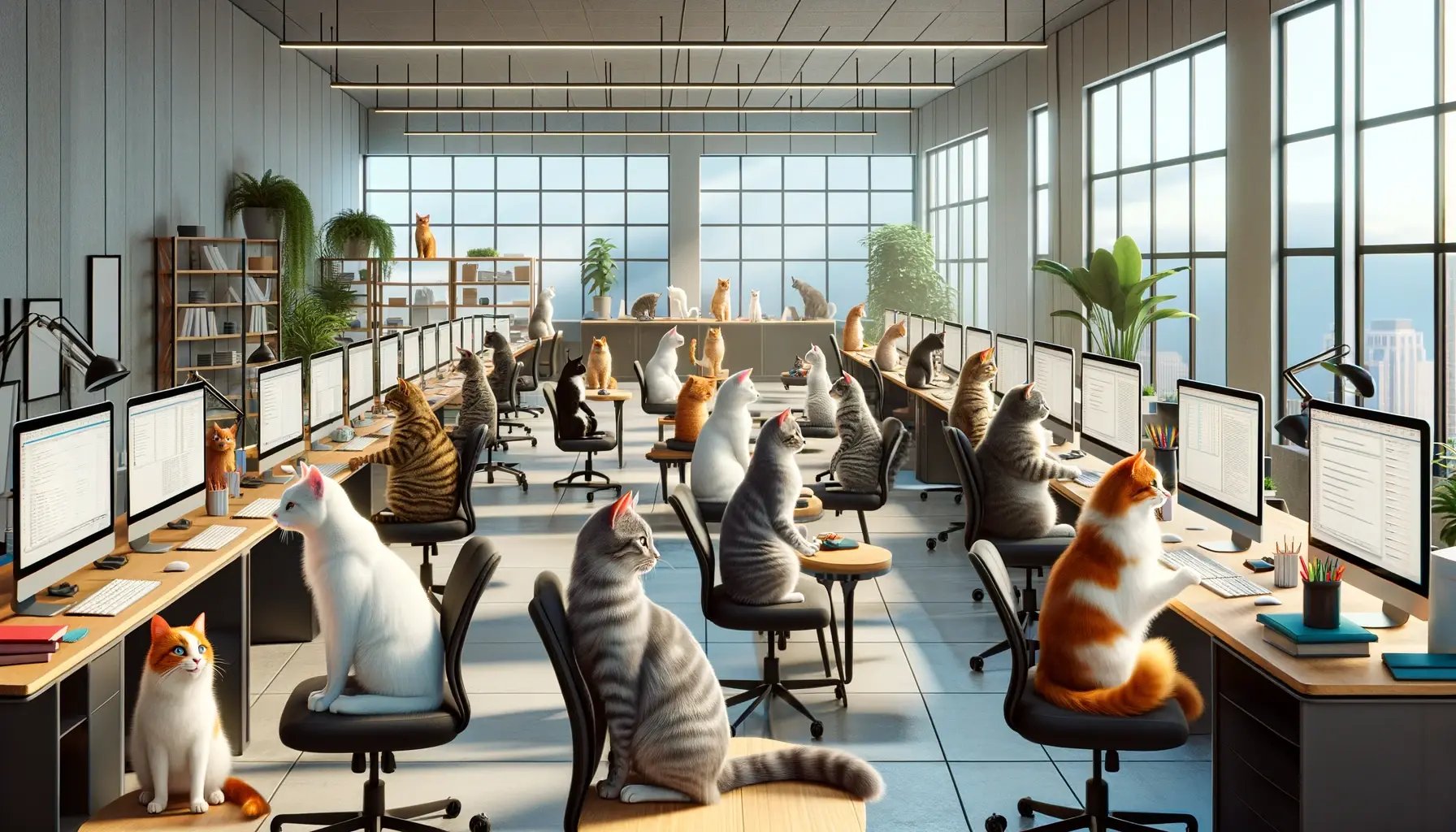
I wanted to see the cats in glasses, so I added that to my prompt and it gave me this:
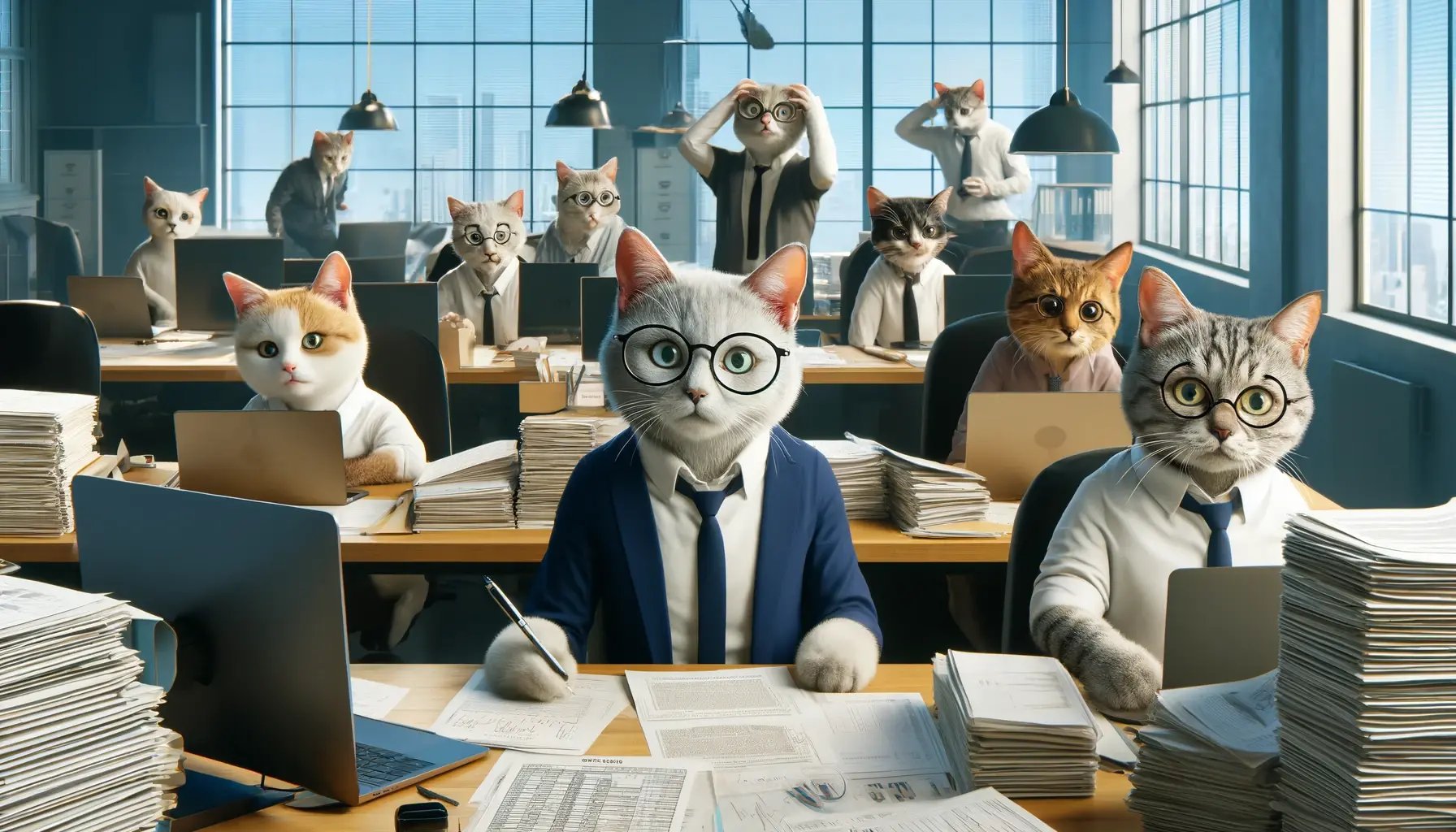
Next, I asked it to make them cuter:
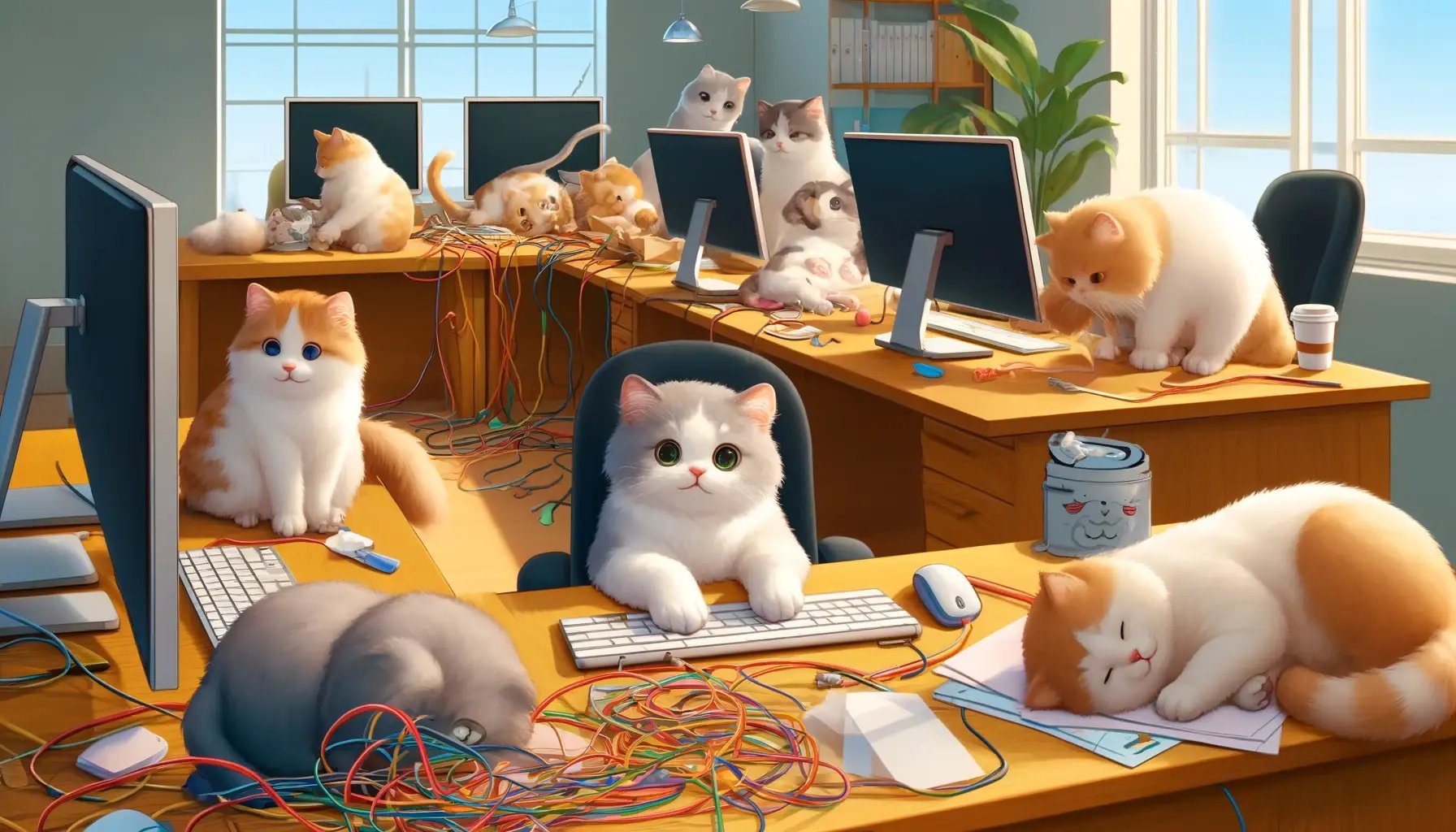
Finally, I asked to make them more corporate, but keep the cuteness and I ended with this image:
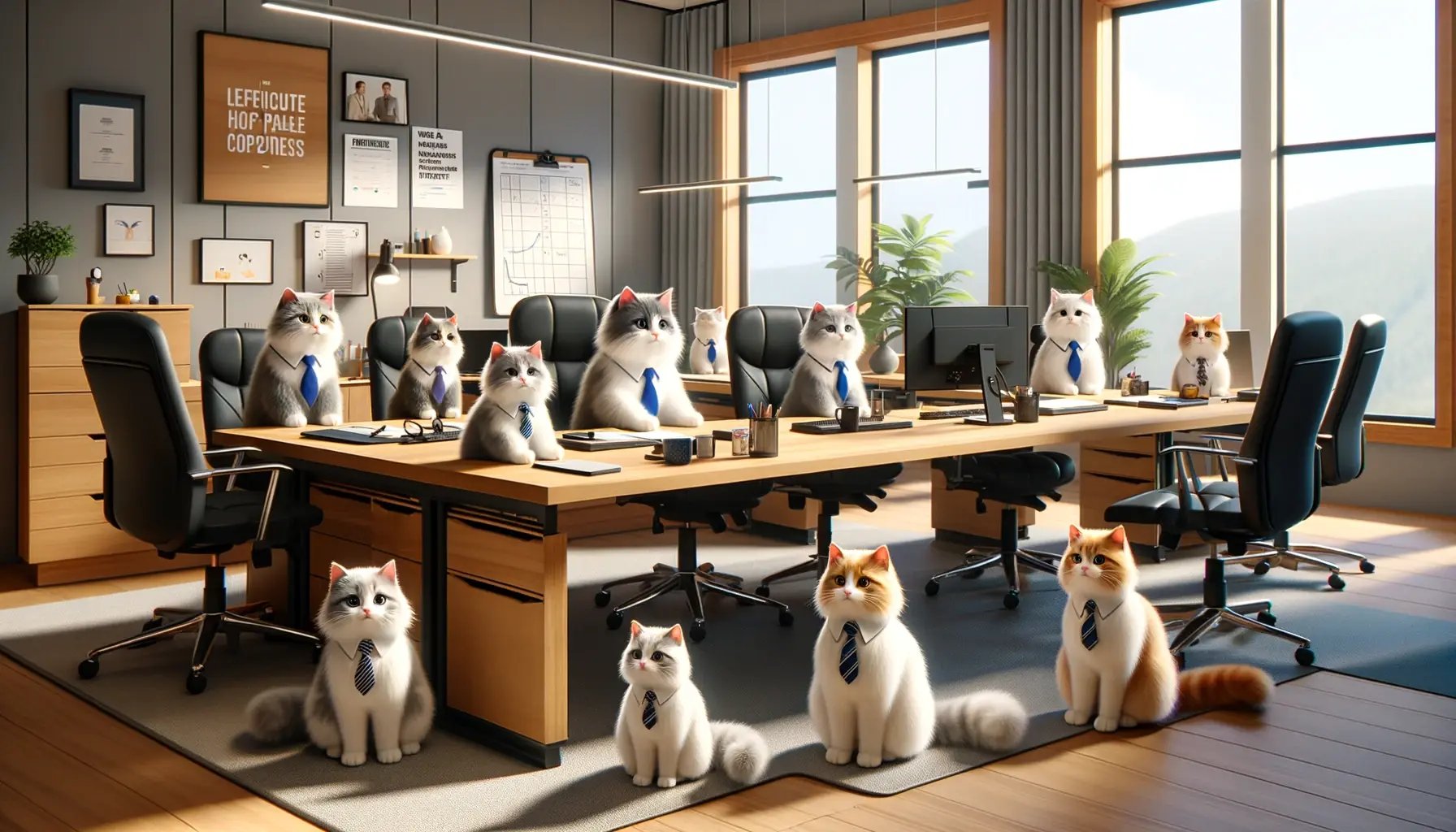
I, of course, could have been more specific and detailed in my requests, but you get the idea, and while this may have been a silly exercise to demonstrate the uses of Generative AI, it can also handle impressive workloads like:
- Writing a proposal
- Doing research for a trip/creating a travel guide
- Generating computer code
- Creating A/B Testing Options
- Etc.
Generative AI can be hugely beneficial but has also made headlines for fears around deepfakes and replacing creative jobs. Later in this blog, we will touch on ethical AI and how it ties in.
Some of the critical benefits of Generative AI are:
- Enhanced productivity
- Increased output of content
- Optimized operations
Natural Language Processing (NLP)
Natural language processing combines computational linguistics with statistical and machine learning models, enabling computers and digital devices to recognize, understand, and generate text and speech.
Human language poses a significant challenge for software development. Factors such as homonyms, homophones, sarcasm, idiomatic expressions, metaphors, grammatical quirks, and variations in sentence structure contribute to this complexity. While humans naturally acquire language skills over the years, programmers must meticulously train natural language-driven applications to accurately recognize and comprehend these nuances to ensure their effectiveness and utility.
NLP is important in how ChatGPT works; it processes the language we input and outputs usable humanlike text. GPT-3 was the first large language model, enabling it to perform even more advanced tasks.
The primary advantage of NLP lies in its enhancement of human-computer communication. While the conventional means of directing a computer involves coding in its language, NLP facilitates a more intuitive interaction by enabling computers to comprehend human language. This capability significantly streamlines the communication process between users and machines.
Some key benefits of NLP are:
- Improved accuracy and efficiency of documentation
- Enables personal virtual assistants and chatbots to communicate and comprehend more effectively
- Quality analysis of structured and unstructured data
Ethical AI
As I have pointed out, AI is becoming increasingly important in society. As with any innovation, ethical boundaries are needed. AI ethics are a guiding principle for those using AI to help define boundaries and ensure AI is used responsibly.
As it stands, there is no wide-scale governing body to determine the ethics of AI, so there is a lot of room for interpretation as people decide how to use the tools available.
Some of the ethical considerations when using AI are as follows:
- Bias Mitigation: AI systems should be designed and trained to minimize biases, ensuring fair and impartial outcomes across diverse populations.
- Privacy Protection: Safeguarding user data and privacy is paramount. AI applications must adhere to stringent data protection regulations and implement robust security measures to prevent unauthorized access or misuse of personal information.
- Accountability and Responsibility: Clear lines of accountability should be established to ensure that individuals or organizations are held responsible for the actions and consequences of AI systems.
- Human Oversight and Control: Human oversight and control should be maintained over AI systems to prevent unintended consequences and uphold ethical principles.
- Environmental Impact: The environmental impact of AI technologies, including energy consumption and carbon emissions, should be considered, and efforts should be made to mitigate negative environmental effects.
- Beneficence and Non-maleficence: AI applications should be designed to maximize benefits while minimizing harm to individuals and society.
- Informed Consent: Users should be provided with clear information about how AI systems will use their data and given the opportunity to provide informed consent for data collection and processing.
- Continual Evaluation and Improvement: Ethical considerations should be integrated into the entire lifecycle of AI systems, with regular evaluations and updates to ensure alignment with evolving ethical standards and societal values.
While legal regulations establish a baseline of acceptability, ethical AI sets higher standards that surpass legal requirements. These standards are designed to uphold fundamental human values and principles, ensuring responsible and conscientious use of AI technology.
Many companies, like Microsoft and Amazon, have created toolkits to help people use AI ethically. Ethical use of AI is paramount in ensuring ongoing innovation progresses our society, not harms it.
As I stated at the start of this, these are the fundamentals for AI. There is much more to know and more to do, so take what you have learned here and run with it. If you want to learn how AI might benefit your business, contact info@concertidc.com today to get started.
Want to Learn How ConcertIDC Can Help Your Business?
Sarah Grace Hays
Marketing Director